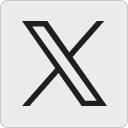


AI-enabled technologies have captured the imagination of every organization. Organizations (both solution providers and buyers) are rushing to jump on the wave of adopting and integrating AI.
Indeed, AI-enabled technologies have already found their way into IT support. An AI-enabled chatbot of today makes its predecessor chatbot of just a few years ago look… well, archaic. AIOps solutions have increased the IT support organization’s observability capabilities by bringing disparate sources of real-time operational data into a single view, facilitating more proactive actions and automated responses when predefined conditions are met.
But one of the challenges exposed by this initial wave of AI adoption within IT support organizations is the inadequacy of its approach to knowledge management. AI-enabled chatbots and AIOps solutions need both data (lots of it!) and organizational knowledge (lots of this too!) to be effective for use.
Knowledge Management (KM) is a key factor in an organization’s capability for being responsive, for driving efficiency and effectiveness, and for making the best use of limited and precious human resources. I believe that effective KM provides organizations with the capability to adapt, shift, change, and respond appropriately, especially in today’s unpredictable and ever-changing business and technology environment.
But many organizations have found that their KM practices aren’t enabling such a capability. Contributing to this situation are a few factors.
- Knowledge becomes stale very quickly – if not maintained. The business and technology environment are continually changing. Stale knowledge is not just “stale” – it can be just flat-out wrong, making it unreliable and worthless.
- In many organizations, it is the IT department that is trying to capture, develop, manage, and use knowledge. Even worse, in many IT departments, it is just the service desk that is investing effort into knowledge management. And many of those service desks, knowledge articles are just a defense mechanism, developed in response to (irate) user demands.
- IT-authored knowledge articles are usually written in “geek-speak” and often read like a technical manual. Such articles are not helpful with enabling consumers to self-service or self-resolve any technology-related issues.
- We (IT) just aren’t that good at writing – not just knowledge articles, but anything that doesn’t resemble application code or scripts.
Enter GenAI
Could the use of GenAI as part of an organization’s KM practices be the low-hanging fruit that delivers the transformational return that organizations need?
Generative AI, or GenAI, are algorithms that can be used to create new content.[i]
GenAI adoption has huge potential to address both the challenges in current approaches to KM, as well as enable organizations (not just IT or the service desk) to better capture, manage, and use its collective knowledge. How could GenAI address the challenges organizations have with KM?
- Overcome that writer’s block. Writing knowledge articles is often viewed as “extra work.” Moreover, those that feel that they are not good writers tend to avoid documenting knowledge in the moment. Using GenAI capabilities and its use of LLMs (Large Language Models), first drafts of knowledge articles can be developed, based on what is entered into systems of record, prior LLM training, and prior curated knowledge articles.[ii] This draft can then be reviewed by experts before being published for use.
- Finally, self-service! The conversational capabilities of GenAI can replace the cumbersome “search and try it” approach with a conversation-like interaction for self-service. Conversation like responses create a compelling pull for the customer; when it works how they expect it to and gets them back to doing their work more quickly, they will return to using self-service.[iii]
- Keeping knowledge fresh. Perhaps the most significant challenge of KM is keeping knowledge relevant and current, regardless of where knowledge is created. Frankly, organizations cannot afford to appropriately hire enough staff to perform this critical, yet often tedious, work. Using the machine learning capabilities of GenAI, new knowledge can be created by combining and synthesizing information from various sources.[iv]
- Making KM an organizational capability. Organizations have long emphasized creating and maintaining documentation, from topics ranging from processes, policies, governance requirements, security, products, applications, and more. There is a wealth of information in different formats for specific needs. LLMs excel at transforming data from one state into another. In the knowledge management use case, this means enabling any knowledge worker to be a knowledge-creation expert.[v]
Warning – challenges ahead
With all the hype and early success around GenAI, it is understandable that an organization may develop a bit of FOMO (Fear Of Missing Out) if they’ve not started adoption. However, FOMO-driven initiatives rarely return any of the expected benefits, and often become money-pits. What challenges do organizations need to address before considering GenAI adoption?
- Ethics and Integrity. Successful implementation will require a focus on ethics, privacy, and security. Guardrails within services and tools as well as ground rules for acceptable use will separate enterprise success from low-level experimentation. From the IT service desk to the software development pipeline and even outside of IT, generative AI is positioned to impact the way work gets done.[vi]
- Data Governance. Organizations must realize that when it comes to GenAI and its use of LLM that “Garbage In” results in “Garbage Out” (GIGO). GenAI responses will only be as good as the data that is used to train the AI. Most organizations lack actively defined and enforced data governance policies.
- Infrastructure impact. The algorithms behind AI are quite complex. LLMs require more computer power and larger volumes of data. The more data available, the better the training of the AI and its associated models. The more parameters defined within a model means the more computer power required. [vii] Investments in infrastructure will be required. AI complexity – LLM require more computer power
- It’s not just about ROI or cost-cutting. It can be extremely easy to look at the introduction of AI-enabled technologies simply as a way to cut costs, reduce headcount, or increase ROI. AI-adoption requires investment, training, and competent people to have success, so view GenAI-adoption success in terms of reduced costs or reduced headcount. Increasing ROI sounds good but measuring ROI (as with most things technology-related) is often difficult. Success metrics such as scalability, ease of use, quality of response, accuracy of response, explainability, and total cost of ownership[viii] should also be considered.
Get ready for GenAI-enabled KM
As with any emerging technology, GenAI presents potential opportunities and capabilities for many organizations. Here are some suggestions for getting ready for GenAI.
- Learn. Most every GenAI solution provider offers no-cost learning opportunities through webinars and publications.
- Review current KM-enabling policies and strategy. What is working well in the current approach to KM? Where are there gaps and resistance? What are knowledge consumers saying about their interactions with knowledge bases? Answers to these questions provide a base for evaluating GenAI solutions for KM.
- Identify areas where improved KM can impact organizational objectives. Identifying how improved KM capabilities can have a positive impact on organizational strategy and objectives is a critical first step in developing a strong business case for GenAI.
GenAI can provide a means for addressing many of the challenges organizations (not just IT) face with its KM practices. It may just be the key to success for the modern organization in the ever-changing digital world.
[i] https://www.mckinsey.com/featured-insights/mckinsey-explainers/what-is-generative-ai , retrieved January 27, 2024.
[ii] https://www.forrester.com/blogs/knowledge-management-id-like-to-introduce-my-new-friend-generative-ai/, retrieved January 22, 2024.
[iii] Ibid.
[iv] Ibid.
[v] Ibid.
[vi] https://www.ciodive.com/trendline/generative-ai/404/?utm_source=CIO&utm_medium=1-2BlastJan18&utm_campaign=GeneralAssembly, retrieved January 22, 2024.
[vii] https://www.ml-science.com/exponential-growth, retrieved January 23, 2024.
[viii] https://ciodive.com/trendline/generative-ai/404/?utm_source=CIO&utm_medium=1-2BlastJan18&utm_campaign=GeneralAssembly, retrieved January 23, 2024.
Share
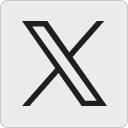


Doug,
Great article!
I agree that KM will be a key to future success. Taking a hard look at your service management system’s ability to manage the ever-changing routines of the enterprise is also a critical enabler.
I’ve been focused on an integrated, non-redundant process model with 5 processes and a simple set of 8 workflows that serve as templates for all daily routines in any service providers’ practice. The management of these routines can be considered the core of the USM method.
This ‘social circuitry’ will be an important, human element, of Gen-enabled KM. I’d love to talk to you about the USM method sometime and get your thoughts.