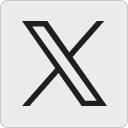


In her book[i], Dr. Kavita Ganesan suggests that any AI adoption be evaluated using three pillars:
- Model success – Is the AI model performing at an acceptable level in development and production? (In other words, the model performs at the required levels of accuracy, execution time, and other factors.)
- Business success – Is AI meeting organizational objectives?
- User success – Are users satisfied with the AI solution and perceive it to be a valid solution?
Many organizations are rushing to incorporate AI-enabled technologies to improve their service management capabilities. AI technologies, such as AI-assistants, chatbots, intelligent process automation, generative AI, and more, can provide a next-level set of capabilities for service management. But are these organizations’ service management practices positioned to fully take advantage of these new capabilities?
Let’s be clear – AI is not a “magic wand.” AI is a technology. And like any other technology, there are factors that must be addressed if an organization is to realize the benefits that AI can bring to service management.
First, AI needs data – and lots of it. The effectiveness of AI depends on the quantity, quality, relevancy, and timeliness of the data being used by the AI models and algorithms. Any limitations in the data being used by AI will be reflected in the outputs produced by AI – and the use of those outputs by service management processes. The old axiom remains true – garbage in will result in garbage out.
AI cannot be a solution looking for a problem. Just because AI is a “hot topic” now doesn’t mean that it is the solution for every business challenge – especially service management issues. If issues like ineffective workflows, undefined services, poorly defined measures, lack of continual improvement practices, or the absence of high-quality data already exist within the service management environment, the introduction of AI will only exasperate those issues.
Lastly, the use of good organizational change management practices is critical. There is a lot of FUD (Fear, Uncertainty, and Doubt) surrounding the introduction of AI[ii] within organizations. Yes, there will be impacts to how humans work and interact with technology, but for whatever reason, there is a heightened fear associated with AI-adoption within service management.
Applying the 3 pillars for AI success to AI-enabled Service Management
Before rushing into incorporating an AI solution with a service management environment, let’s adapt and apply Ganesan’s three pillars for success with AI-enabled service management.
The first pillar is business success. How do current service management capabilities support business outcomes and enable value realization? How will the introduction of AI capabilities further enhance the realization of the outcomes and value delivered by service management? If the answers to the above questions aren’t clear, revisiting some foundational elements of service management is in order. Consider the following:
- Have IT services been defined, agreed, documented, and measured in terms of business value, business outcomes, and the costs and risks associated with the delivery of services? Many IT organizations have defined what they call “services” in terms of
- what goods and products (like laptops and smart devices) are provided
- the service actions (like password resets) a service desk will perform, and
- procedures for gaining access to digital resources (like a cloud-based resource or a shared drive).
Not only does this approach inhibit a mutual understanding of the vital role of technology in business success, but it also commoditizes what IT does. Secondly, this approach fails to establish business-oriented measures regarding results and value.
- Are non-IT colleagues named as service owners? Are these non-IT colleagues actively involved in the delivery and support of services? This is a significant issue for many service management implementations. In many organizations, IT personnel, not non-IT colleagues, have taken on the role of service owner – the person that is accountable for a service meeting its objectives and delivering the required business outcomes and value. The service owner is critical to understanding what is needed and importantly, how business outcomes and value are realized and should be measured.
- How might AI adoption enable organizations to consider service management practices that would enhance their business? For example, better service portfolio management would enable better utilization of and data-driven investments in services and technology.
The next pillar is employee success. Frequently (and counterintuitively!), service management practices have been designed and implemented with IT and not the IT service consumer in mind. As a result, interacting with the service desk or a self-service portal can be an exercise in frustration due to the over-technical nature of those interactions. Consider:
- How might the introduction of AI result in friction-free interactions with services and the fulfillment of service requests? How might AI personalize end-user interactions with service management practices? Consider how AI could shift the burden of interacting with service management practices from the end-user to a personalized and proactive AI-enabled capability.
- How might the introduction of the AI model result in friction-free interactions with supporting IT services? If consuming IT services present challenges to end-users, it can also be challenging for those that deliver and support those services. Will AI-capabilities enable service management practices to shift from a reactive to proactive stance by identifying and eliminating causes of incidents before they occur? Will AI-capabilities enable better issue resolution by suggesting potential solutions to IT technicians?
- How might the introduction of AI enable employees to make better, data-driven decisions based on relevant, timely, and accurate knowledge? Knowledge management is among the most significant challenges of a service management implementation, as knowledge is ever evolving and continually being created, revised, and applied. AI may provide a solution – this blog explores how Generative AI could provide organizations (not just IT) with the capability of harnessing its collective knowledge.
The final pillar is AI / service management model success. Frankly, many service management challenges can be resolved through continual improvement activities. Some issues may be resolved through the application of effective and efficient automation. Questions to consider include:
- How might AI adoption result in better and proactive detection and resolution of issues before those issues impact the organization? How might AI adoption result in improved change implementations through better testing or confirmation of positive business results?
- Is there sufficient, good-quality data to enable AI-driven service management actions? If AI models are not supplied with sufficient, good-quality data, the results from the model will be suboptimal at best – or worse, just flat-out wrong.
- What is the required level of accuracy for the model? A “100% accurate” model may be too costly to achieve and maintain; a “75% accurate” model may be perceived as a failure.
Get ready for AI-enabled service management
The introduction of AI to a service management environment can be a game-changer on many levels. Here are four steps to get ready:
- Make the business case for introducing AI to service management. Think strategically about AI , service management, and how the combination of AI and service management will help the organization achieve its mission, vision, and goals.
- Communicate, communicate, communicate. The mention of AI adoption may cause concerns among employees. Start open conversations regarding AI-enhanced service management capabilities, incorporate feedback, and proactively address concerns.
- Identify and define success measures. The mere implementation of AI capabilities within service management is not an indicator of success. Define how the benefits articulated in the business case will be captured, measured, and reported.
- Begin data governance now. The success of any AI initiative depends on the availability of good quality data. If service management is to leverage AI capabilities, the data being captured must be of good quality. Define and publicize data quality standards for service management practices and ensure compliance through periodic audits.
The introduction of good AI capabilities will not fix bad service management. Applying the three pillars described above will ensure successful introduction of AI capabilities resulting in next-level service management practices for any organization.
Is your service management approach “AI-ready”? An assessment by Tedder Consulting will identify any foundational gaps so your service management environment is “AI-ready”. Contact Tedder Consulting today for more information!
[i] Ganesan, Dr. Kavita. “The Business Case for AI: A Leader’s Guide to AI Strategies, Best Practices & Real-World Applications”. Opinois Analytics Publishing, 2002.
[ii] https://www.forbes.com/sites/jenniferfolsom/2024/03/28/meet-your-newest-co-worker-ai Retrieved April 2024.
Share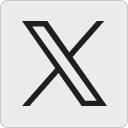

